“The true sign of intelligence is not knowledge but imagination” – Albert Einstein
Who should read this book: CEOs, executives, and those looking to improve their businesses to gain competitive advantages with artificial intelligence.
I regularly review AI books and highly recommend Prediction Machines. This book is unique because it delves into applied-AI use cases versus simply the technology itself. The authors’ focus is how to identify and re-engineer business decisions that make up a company’s work stream in order to capitalize on the exploding amount of data, and the rapidly falling cost of prediction technology. After working with emerging growth companies in predictive analytics and Applied AI for the past 25 years, experience has shown that re-engineering work streams is the key bottleneck for companies that will benefit from the rapidly growing pool of AI technologies and services available in the market today.
The Authors’ Big Ideas
- As developments in AI advance, prediction becomes less expensive and improves. Moving from 90% to 99% is not 10% better, it’s actually 10 times better because the change in the error rate and this order of magnitude change will create new opportunities.
- Improving decision making in workstreams is the first step to implementing Applied AI. Better predictions will change how companies approach challenges. The benefit is that workflow re-engineering is minimized. Decision trees as shown in Figure 8-1 are a good tool to deconstruct an Applied AI work process.

The authors use credit card fraud detection as an example.
The way credit card companies define the problem and set the rules determines how much loss from fraud they have or how dissatisfied their customers may be for rejecting legitimate transactions (Type 1, Type 2 Error). Data on purchase behaviors and history, help the algorithm improve human judgement, but the process is not purely algorithmic since exceptions occur that do require human judgement. The level of customer satisfaction versus fraud loss tolerance will be a strategic decision here and will require human judgment in setting up the model and how to manage exceptions.
- The key components to making applied AI work are robust data, multiple outcomes and a feedback loop on outcomes for the predictions as shown in Figure 7-1.

- Machines are strong in data intensive environments but fall short at properly managing exceptions. Humans are stronger when there is little or poor data, and they must operate on a hypothesis. Often, the optimal solution for many AI applications is human-assisted AI. AI predicts what is in the range of high confidence and humans oversee and handle exception events.
How to apply this book
At Savant Growth, we often ask our portfolio companies’ CEOs what they would like to predict if they have the perfect data or information. In order to grow faster and be more successful, a key factor for many of our companies is reducing Customer Acquisition Cost (CAC) and accelerating CAC payback—or how quickly companies recover the cost of acquiring customers.
We developed SaleSavant to help our portfolio companies address and reallocate time to prospects with the quickest paybacks. The next step for companies is to revisit all their workflows to identify how multiple work streams can be optimized in parallel with data and better predictions to potentially re-engineer an entire service offering. As an example, for our product led growth portfolio companies, SaleSavant helps identify high value prospects (whales) from this stream of inbound visitors that are based solely on their web signatures to prioritize and customize how they are handled to improve close rates.
The companies take advantage of AI similarly will have the greatest opportunity for disruption as shown in Figure 12-1. The resulting process changes can be dramatic: we envision that the auto dealer of tomorrow will be able to predict when a prospect is ready for a new car and the likelihood to purchase accurately enough that they can offer the prospect a free test drive for a week from the convenience of their driveway.
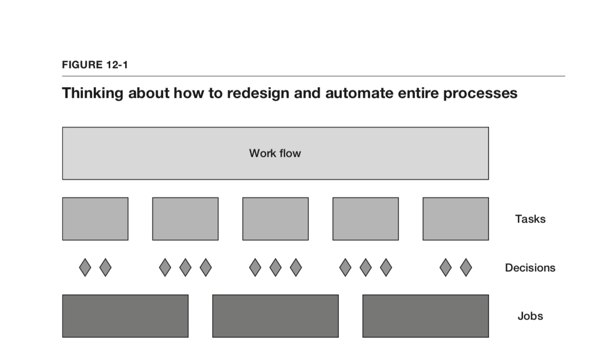
We believe the redesign of workstreams in every aspect of business will create exciting new, market-leading companies. We are actively investing in this space and looking to integrate Applied AI wherever possible into our portfolio companies and our own investment activities. We are eager to see how this technology and related markets develop and learn from the tremendous innovation that is underway.
Add more specific examples. E.g. “by doing XYZ we managed to get our CAC down with 30%, resulting in x-% higher conversion”- Olivier
About the Authors of Prediction Machines:
Ajay Agrawal, Joshua Gans, Avi Goldfarb are Professors of Strategic Management and Marketing at the University of Toronto’s Rotman School of Management. Agrawal is a founder; Gans is Chief Economist; and Goldfarb is Chief Data Scientist at the University of Toronto’s Creative Destruction Lab. Additionally, Agrawal cofounded the AI/robotics company Kindred, with the mission of building machines with human-like intelligence.